Research
Most information in today’s society is being produced, processed and stored digitally. The world has just witnessed the power of digitized information dissemination via Twitter during the Arab Spring, the Haiti earthquake and the Fukushima disaster, facilitating the uprising, organization and spread of information. However, we know very little about the functions of information dissemination and the trigger mechanisms offered by language. Information is never neutral but always couched in a context of viewpoints, beliefs, rumors, evidence, certainty and uncertainty. The aim of this interdisciplinary project is to account for stance in language use, to develop computational methods for its identification and description and finally, to produce interactive visualizations that are capable of communicating the data through formats other than strings of text.
We focus on web-based streaming data sets (e.g., websites, blogs, forums, or microblogs) provided by Gavagai as primary data source. This kind of streaming text data is time-stamped, arrives in real-time, and is highly dynamic, i.e., it changes size and complexity constantly.
The StaViCTA project was performed from 2013 until end of 2017. The complete list of publications based on our research in the project can be found here.
Linguistics Group at Lund University
Members: Vasiliki Simaki (affiliated to LNU) and Carita Paradis
Stance-taking in human communication is the expression of attitudes and opinions, and as such it is crucial for the social construction of meaning in discourses of different kind. At the same time as expressions of stance are pervasive in language, they are often not immediately observable to language users, since they do not form a uniform grammatical or lexical category. Stance is expressed through a cascade of forms. Some of them are easily identifiable constructions, such as ’I don’t know but . . . ’, ’we think’, ’perhaps’, ’surely’, ’must’, ’likely’, or ’believe’, while other expressions are more hard-to-get-at stance marking as in: ’I am not your maid’, ’It is what it is’, ’Couldn’t be bothered’, ’We are really feeling really pissed off ’, ’If you don’t have an iPhone I feel bad for you son’.
It follows from this that our point of departure was to explore stance from a notional point of view and treat utterances that express speaker stance in a holistic way. Stance categories were identified on the basis of utterance meaning and not on the basis of individual words. For this purpose, ten core stance categories were derived from the literature, and a cognitive-functional framework was designed. The stance concepts used in our research were AGREEMENT/DISAGREEMENT, CERTAINTY, CONCESSION/CONTRARINESS, HYPOTHETICALITY, NEED/REQUIREMENT, PREDICTION, SOURCE OF KNOWLEDGE, TACT/RUDENESS, UNCERTAINTY and VOLITION. These categories were used as annotation labels for our corpus from blog posts: the Brexit Blog Corpus (BBC).
This notional annotation procedure aimed to determine how speaker stance is communicated through language instead of through a pre-selected list of words, which of the categories are used in natural language use where, how, how often, and it was shown that some categories tend to combine with one another and support and reinforce one another, while others do not. For instance, through the utterance For sure, there will be showers in Wales tomorrow the speaker makes a PREDICTION about the weather, and it is also clear that the speaker presents the information with a high degree of CERTAINTY in spite of the fact that predictions of this kind are sometimes not that reliable or not certain at all. The annotations were then used to feed into the work of the computational development and the visualization of the data and the final goal was to describe and explain how speakers use stance in text and discourse. We also subsequently used the annotated data to explore the utterances from a lexical point of view in order to determine what words and constructions were used to express the different stances in order to create profiles of the lexical instantiations of stance in text and discourse.
Computational Text Analytics Group at Gavagai AB
Members: Maria Skeppstedt (affiliated to LNU) and Magnus Sahlgren
Computational text analytics usually involves processing large amounts of text in order to make an utterance understandable by computers. To achieve this goal we will use insights from the linguistics group about the language structures that are used in stance taking. We will also use various computational linguistics/NLP approaches to extract those linguistic features automatically. These features are then fed to machine learning methods to find out what an utterance means and what kind of stance the speaker intends to take. Thus, the main research questions concern how to extract linguistic features related to stance taking, what machine learning methods are suitable for detection of stance, and how annotated data to train stance classifiers can be created efficiently. With huge amounts of data available in digital text media, it is necessary for all of the processing modules to be 100% scalable. The information extracted by the text analytics unit can then be used for visualizing the overall process of stance taking in digital text media.
Our research includes an evaluation of different kinds of feature sets for sentiment and stance classification. We have used bag-of-words features, higher order n-grams, distributional semantics features and also the trending deep learning methods for unsupervised feature learning. We have also explored the possibility of reducing the amount of manually annotated data that is required, by applying active selection of relevant training samples for the classifiers. The experiments have been conducted on sentiment polarity data sets and data sets in which some components of stance taking, such as 'uncertainty', 'hypotheticality', 'contrast', 'agreement' and 'disagreement', have been annotated.
Our overall aim is to improve the accuracy and usefulness of sentiment classification and also to be able to process huge amounts of dynamic streaming text data.
Visual Text Analytics Group at Linnaeus University
Members: Kostiantyn Kucher and Andreas Kerren
There is no visual text analytics system especially designed for the visual analysis of stance in large streaming text data. We address the challenging combination of huge amounts of dynamic text data—which also include temporal and geo-spatial dimensions—with the development of a visual text analytics system that allows the interactive visualization and exploration not only of the texts themselves, but also of the vast multidimensional data generated by the machine learning methods used for capturing and analyzing stance-related phenomena.
During the first stages of the project we have implemented a visual analytics system uVSAT for explorative analysis of data provided by Gavagai. uVSAT uses the sentiment analysis approach based on occurrences of stance markers (seed words) in social media texts. It provides interactive visualizations for temporal data, sets of corresponding HTML documents, and detailed text content of such documents. Our partners from the linguistics group have been using uVSAT to get an overview of temporal developments in data, analyse particular document sets in detail, and export new stance marker candidates for further use.
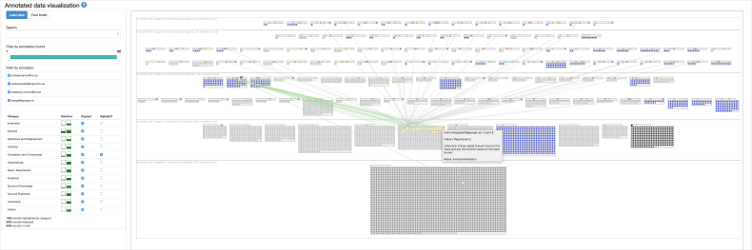
In the main phase of the project, we have been working on a visual analytics system called ALVA, which is designed to support all stages of training for a stance classifier. ALVA supports data annotation, including active learning feedback required for classifier improvement. ALVA provides our experts in linguistics and computational linguistics with a visualization of the annotated data that focuses on the distribution and co-occurence of multiple stance categories. This kind of visual analysis helps our collaborators improve the understanding of stance patterns and, consequently, enhance the classifier.
Finally, we have contributed multiple stance visualization approaches that make use of the classifiers developed by our collaborators. Our most recent visual analytics approach called StanceVis Prime supports sentiment and stance analyses for temporal text data from social media. It allows the users to (1) explore and compare multiple data series (based on two sentiment categories and twelve stance categories, among others) from several data sources (such as Twitter and Reddit), (2) identify regions of interest with large numbers of sentiment and stance occurrences, and (3) investigate the underlying sets of text posts with both close and distant reading supported. Our additional contributions demonstrate further applications of stance analysis and visualization, including the support for (1) geospatial data from tweets with a visualization tool called StanceXplore, (2) longer individual documents such as books and business reports with a tool called DoSVis, and (3) facilitation of analysis of topics and arguments in social media texts with a customized version of a tool called Topics2Themes.
The future work on stance visualization includes development and validation of special representations and techniques to tackle the issues of dynamic (streaming) data, inconsistencies of data availability and granularity, and revealing latent patterns in the data with the scalability concern in mind.